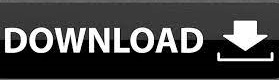
# Read data from a table df = sql_context. sql import SQLContext sc = # existing SparkContext sql_context = SQLContext( sc) Apply some transformations to the data as per normal, then you can use the // Data Source API to write the data back to another table option( "query ", "select x, count(*) my_table group by x ") Can also load data from a Redshift query val df : DataFrame = sqlContext.read option( "tempdir ", "s3n://path/for/temp/data ") option( "url ", "jdbc:redshift://redshifthost:5439/database?user=username&password=pass ") Get some data from a Redshift table val df : DataFrame = sqlContext.read _ val sc = // existing SparkContext val sqlContext = new SQLContext(sc) To use these snapshots in your build, you'll need to add the JitPack repository to your build file.
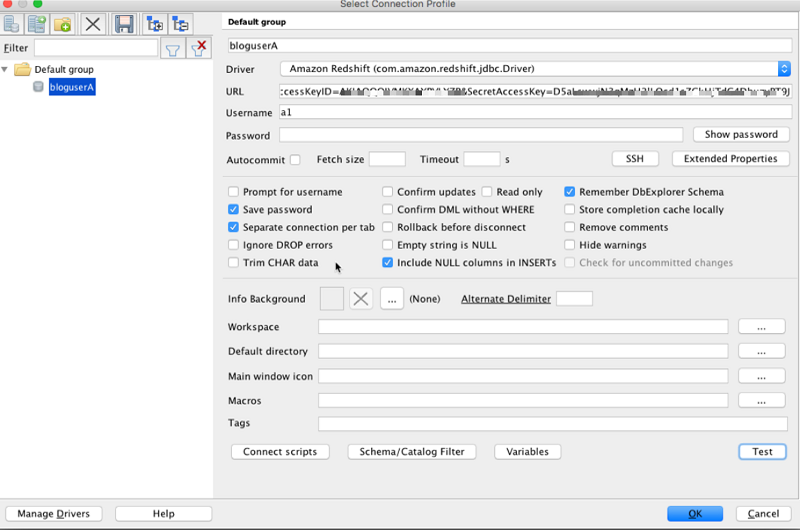
Master snapshot builds of this library are built using jitpack.io. See the comments in project/SparkRedshiftBuild.scala for more details.

However, if you get ClassNotFoundExceptions for Amazon SDK classes then you will need to add explicit dependencies on -java-sdk-core and -java-sdk-s3 as part of your build / runtime configuration. In most cases, these libraries will be provided by your deployment environment.
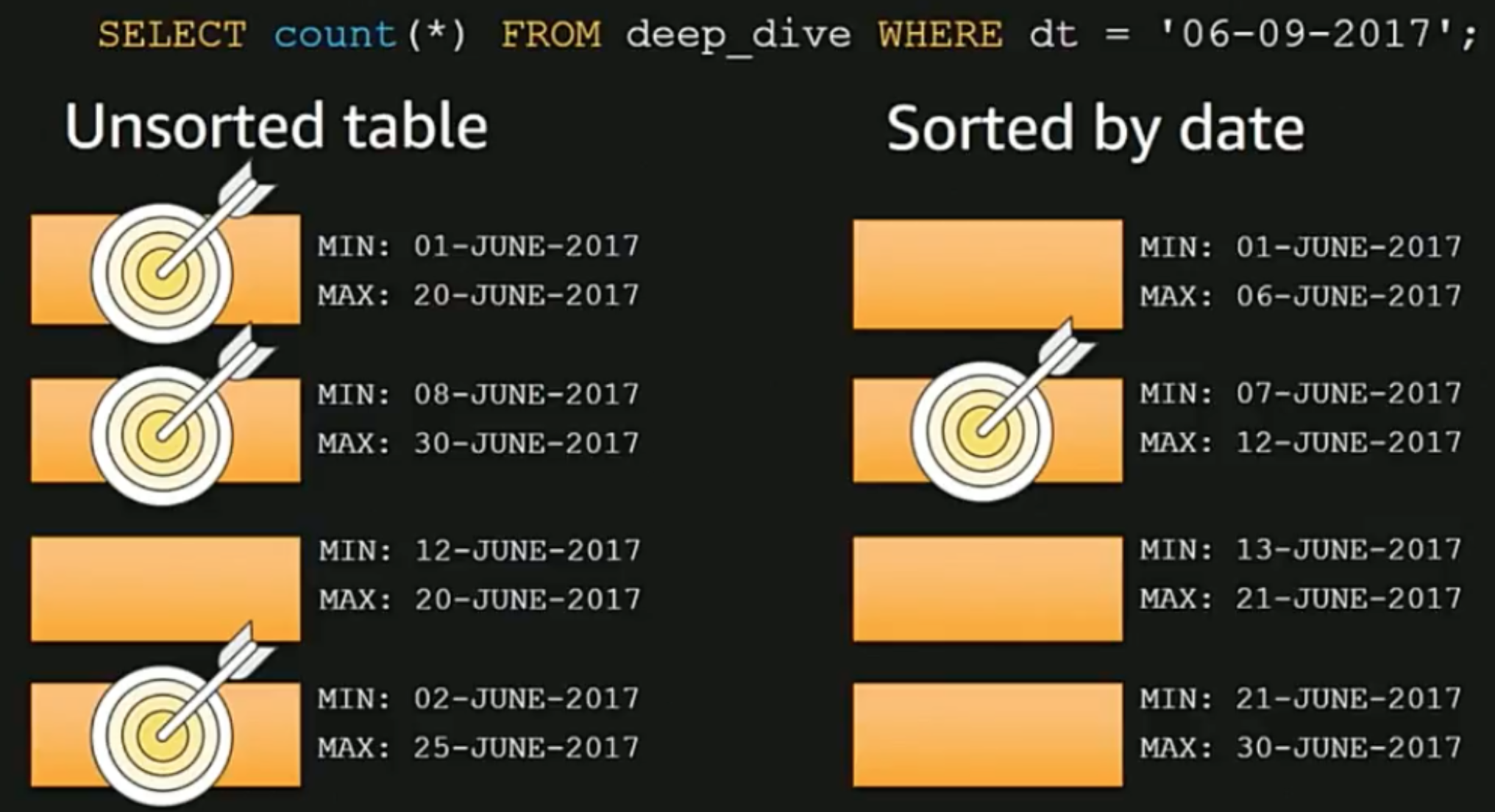
Note on Amazon SDK dependency: This library declares a provided dependency on components of the AWS Java SDK. In most deployments, however, this dependency will be automatically provided by your cluster's Spark assemblies and no additional action will be required. However, you may need to provide the corresponding avro-mapred dependency which matches your Hadoop distribution. Note on Hadoop versions: This library depends on spark-avro, which should automatically be downloaded because it is declared as a dependency. This library has also been successfully tested using the Postgres JDBC driver. Amazon recommend that you use their driver, which is distributed as a JAR that is hosted on Amazon's website.
CREATE TABLE REDSHIFT DRIVER
You will also need to provide a JDBC driver that is compatible with Redshift. You may use this library in your applications with the following dependency information: This library requires Apache Spark 2.0+ and Amazon Redshift 1.0.963+.įor version that works with Spark 1.x, please check for the 1.x branch. S3 bucket and Redshift cluster are in different AWS regions.Configuring the maximum size of string columns.Data sources API: Scala, Python, SQL, R.
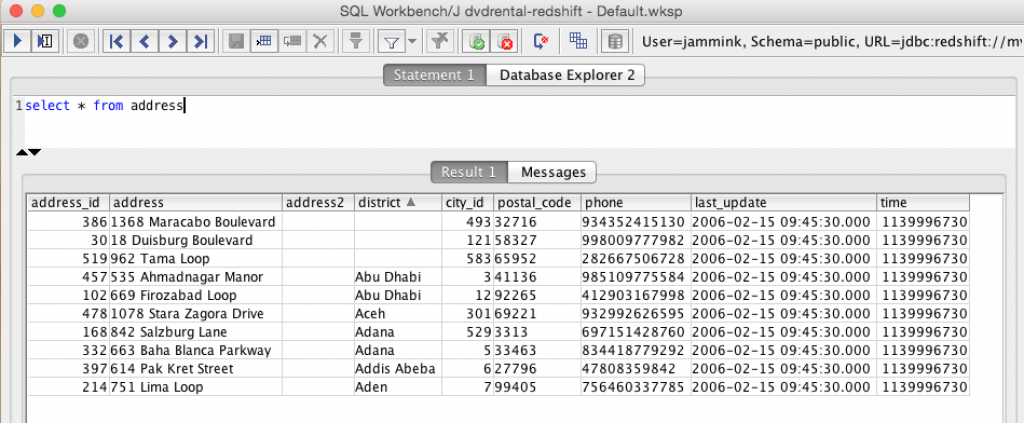
If you plan to perform many queries against the same Redshift tables then we recommend saving the extracted data in a format such as Parquet. This library is more suited to ETL than interactive queries, since large amounts of data could be extracted to S3 for each query execution. JDBC is used to automatically trigger the appropriate COPY and UNLOAD commands on Redshift. Amazon S3 is used to efficiently transfer data in and out of Redshift, and Original ReadmeĪ library to load data into Spark SQL DataFrames from Amazon Redshift, and write them back to As a result, we will no longer be making releases separately from Databricks Runtime. For more information, refer to the Databricks documentation. The latest version of Databricks Runtime (3.0+) includes an advanced version of the RedShift connector for Spark that features both performance improvements (full query pushdown) as well as security improvements (automatic encryption). To ensure the best experience for our customers, we have decided to inline this connector directly in Databricks Runtime.
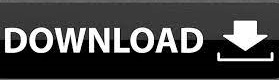